Observations #3
Every Friday morning (only if there is something unique to write about) a set of ideas and observations from a week’s worth of work analyzing businesses and technologies.
Payment models for diagnostics
Molecular diagnostics especially companion tests have been improving rapidly; however, their ability to get reimbursed has been lagging. Moreover, doing long-term economic/health benefit studies for each new test is prohibitive. This is a main reason why Foundation Medicine is such an incredible case study because they figured out how to design a business model to buck the trend. A recent article out of ASCO - https://ascopubs.org/doi/10.1200/PO.19.00210 - explores new payment models and proposes their own where networks guarantee payments for new diagnostic tests regardless of market uptake. The research is a good starting point but probably not practical for every disease - a top-down distortion often leads to large negative outcomes in markets. Usually, bottom-up incentive changes get closer to the right solution.
Diagnostics are incredibly important to guide treatments for patients and help prevent adverse events. Few healthcare networks have really figured out how to become truly outcomes-based and thereby probably reward these tests. There are three issues that need to be addressed and if one network can solve it, healthcare payments are a monkey see, monkey do type of industry so change should come about rapidly:
Portability of coverage across various private plans - this would enable treatment plans to remain consistent
Coupling reimbursement of diagnostic tests to clinical data and following through on the potential payments to incentivize diagnostic companies to build out robust utility packages for clinicians and payors.
Deployment of different types of payment plans - population-level type of coverage for tests and risk-sharing between diagnostic companies and payors.
As the shift toward fee-for-value is occurring especially for private plans, payments for diagnostics will become more reliable as long as they lead to better outcomes for patients and overall populations.
Genetic circuits and computing
A tantalizing review out of Newcastle - https://www.nature.com/articles/s41467-019-13232-z - laid out a concept of cellular supremacy where computation from a cell can outperform a classical computer for a set of algorithms similar to the idea of quantum supremacy. I did not understand some of the review’s arguments about biological computation and how to make it usable, but I found the piece a tantalizing launchpad to think about the prerequisites for useful molecular computers.
Moving beyond the combinatorial logical model - initially, porting models form computer science to biology enabled proof-of-concepts like the repressilator and generated a large set of modular and tunable components
Different ways to measure “reliability and predictability of genetic circuits”
Reduce the amount of actual biological material required to solve large-scale problems, like graph searches of >100 vertice objects, by using the entire suite of biological processes from DNA replication to metabolism to solve problem and automate the actual computation. This is probably the most important point; I’m having a hard time distilling this into a few sentences and not ~10 pages, but the general idea is to better integrate genetic circuits with the chemical/physical properties of cells.
Using biology for computation has been restricted by the current conceptions of computation derived from electronic components. But by relying on a combinatorial framework, molecular computers have always been limited in their ability to truly harness the power of biology to establish its superiority in some situations. A few next steps need to be taken, off the shoulders of the giants of synthetic biology, to begin to program biology and build applications where these types of computers thrive - in highly uncertain environments with a need for complete autonomy.
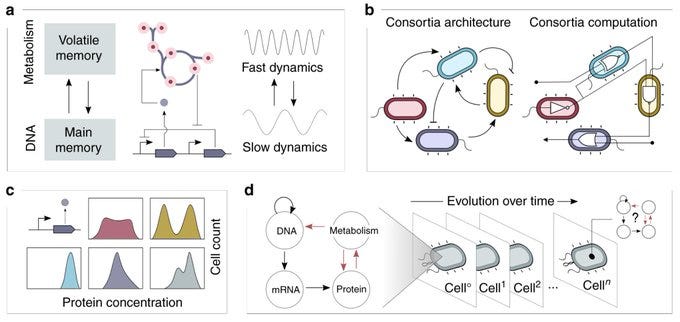
Designing CARs
Out of UCSF, Kole Roybal, one of my favorite emerging inventors, wrote a recent review on the current toolkit to design chimeric antigen receptors (CARs). The piece lays out 4 key bottlenecks:
Tumor specificity and infiltration (potential solution: TILs)
Control of cell activity, proliferation, and persistence (potential solution: a screening platform to what Serotiny has built)
Engineering tumor microenvironment (TME) responsive cells (potential solution: TRUCKs)
Scanning for true tumor-specific antigens (potential solution: neoantigen screening platform similar to what Gritstone or TScan has built)
The current version of CAR-T therapies are probably sufficient to cure many blood cancers. However, to gain complete coverage and pursue solid tumors (the 90% of cancers), CAR designs as well as the underlying biological circuits need to improve. The key insight is that first-generation CARs sought to do its best to mimic T-cell receptor (TCR) signaling; however, CAR design still has been relatively limited - TCRs emerged from “millions of years of evolution” whereas CARs have only been under intense study for a decade or so. Successive generations of CARs have added to effectively what amounts to spiffy add-ons to a hotrod: nice rims, a flame on the side, and a rear spoiler. New domains are being added to CARs across generations to create higher-order capabilities to solve the four bottlenecks Roybal laid out.
Overall with the ability to print increasingly cheaper DNA, analyze a large-set of high-throughput experiments, and modular tools from synthetic biology, an accelerated evolution can be applied to CARs to fulfill a wide set of functions:
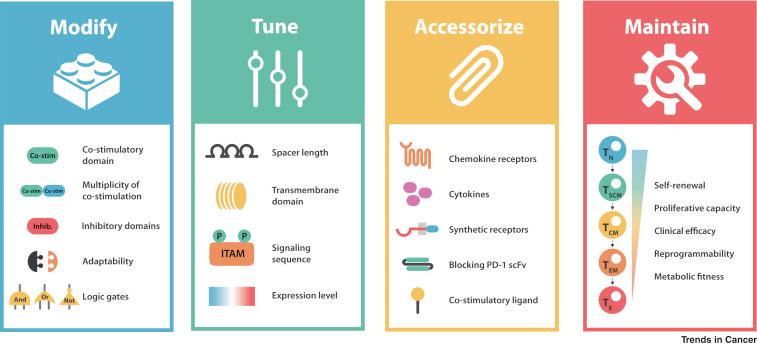
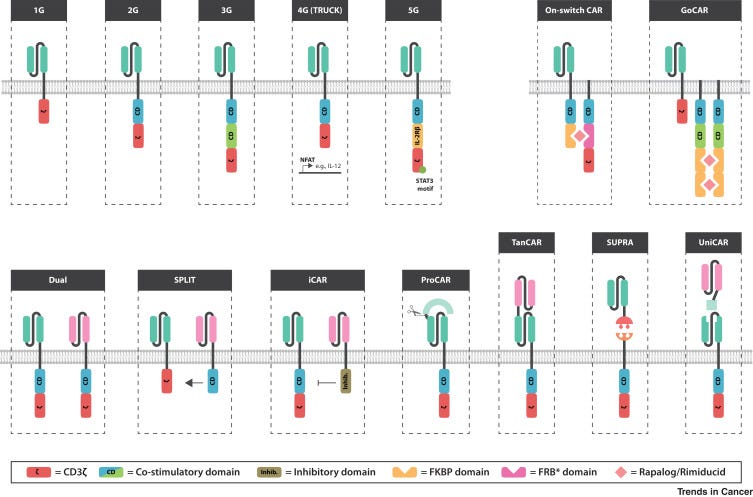
Autoimmunity, heart disease, and infectious disease - what all three have in common?
Companies pursuing all three disease sets need new business models to make a dent in them. Pricing power is low for infectious disease products, the costs for clinical trials in heart disease is prohibitive for almost every new entrant, and autoimmunity is still being treated with blunt tools similar to cancer 20 years ago.
Autoimmunity: clinicians still treat a broad array of autoimmune patients with the same regimens. This is similar to how cancer patients used to be bucketed into very large classes and treated non-precisely. Now cancer patients more often than not have their tumor sequence and a particular cocktail of drugs prescribed to them. Autoimmunity is making a similar transition. New drug companies are emerging to meet this demand as well as new biomarkers and models to stratify subset of say lupus or RA patients.
Heart disease: to show non-inferiority as well as to get the FDA’s approval given the large number of approved heart disease medicines already on the market, companies need a large war-chest to complete trials for new drugs. This limits the amount of creativity in the field where smaller companies often don’t have a chance to bring their own drugs to patients. New models to recruit patients as well as generate control arms need to emerge to accelerate progress for heart disease patients.
Infectious disease: the US healthcare system gives very little incentive to make new medicines for infectious disease. This problem is very acute for antibiotic developers. A well-capitalized business like Vir Biotechnology has a shot given the amount of cash, but most companies do not have that access to capital. New regulations and incentives might help but are a long-shot to change anytime soon. New models especially at the early-stage have been emerging to design companies that generate cash not only from drugs to treat the disease but measure and manage it - a full-stack model.
Versioning medicines
The power of new medicines is rapidly increasing along with their prices. A bigger problem than the price tag is as drugs begin to cure disease starting with blood cancers and viral disease, new business models need to emerge to solve lets call it a cure cliff (similar to a patent cliff). Where once a patient is cured or effectively cured, a drug development company no longer generates revenue. This is incredibly important for patients and the overall healthcare system; however, drug companies need to respond by versioning products either through label expansion or improved efficacy.
Similar to the business model transition that occurred 3-4 decades ago from blockbuster drug development to a rare disease model, this new transition will require drug companies to become fully integrated - not only making new medicines but getting involved in diagnosis and measurement as well as downstream management.
New reimbursement models (i.e. outcomes-based pricing, population coverage) will emerge mainly around pricing with winning drug companies collecting data around real-world evidence, outcomes, and efficacy to inform both their product development efforts as well as marketing.
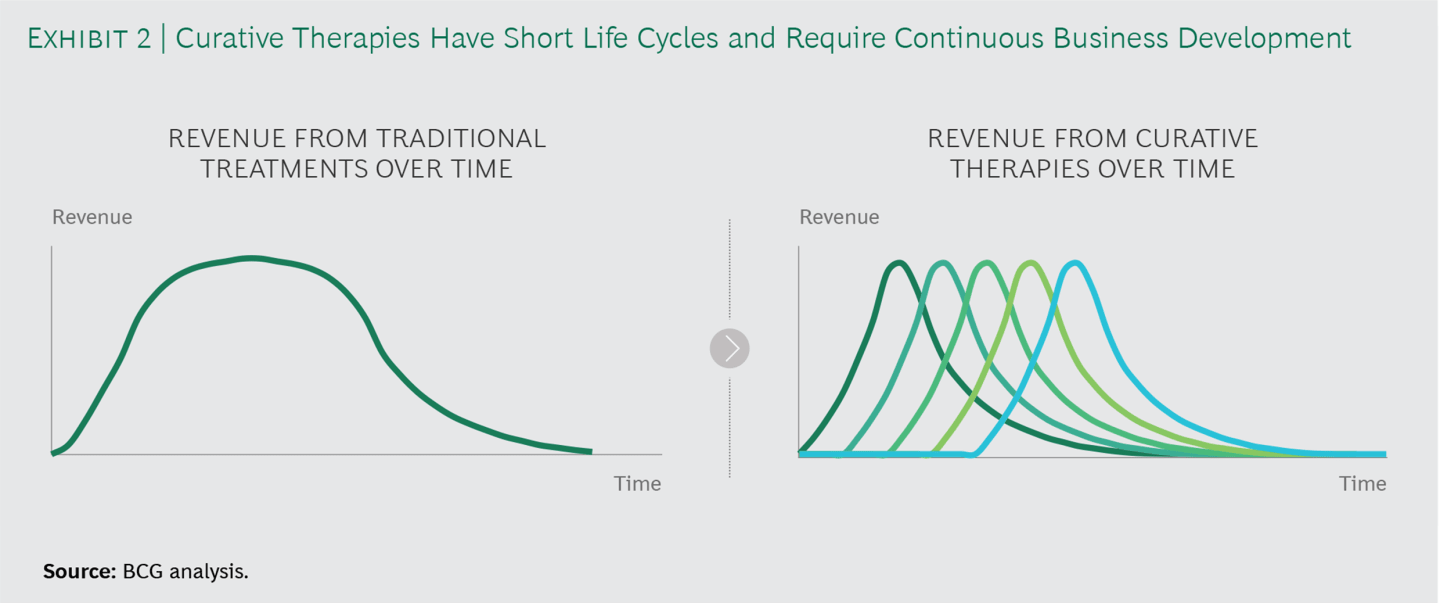